
Precise emotion ground truth labels for 360° virtual reality (VR) video watching are essential for fine-grained predictions under varying viewing behavior. Together, these findings suggest that our two-handed method for two-dimensional continuous ratings is a powerful and reliable tool for future research. The validity of two-dimensional responding was also demonstrated in comparison to one-dimensional reporting, and in relation to post hoc ratings. Results show that our new method is easier to use, faster, more accurate, with reduced method-driven dependence between the two dimensions, and preferred by participants.
#Sc joystick mapper 2.5 series#
In a series of tasks, the study reported here addressed these limitations by comparing a previously used method to a newly developed two-handed method, and by explicitly testing the validity of continuous two-dimensional responses. Third, two-dimensional reports have primarily been validated for consistency between reporters, rather than the predictive validity of idiosyncratic responses. Second, respondents report on two dimensions using one hand, which may produce method driven error, including spurious relationships between the two dimensions. First, current methods are primarily suited for bipolar, as opposed to unipolar, constructs. For all the variety of approaches, several limitations are inherent to most of them. Recent studies have extended continuous self-report methods to simultaneously collecting ratings on two dimensions of an experience. Research on fine-grained dynamic psychological processes has increasingly come to rely on continuous self-report measures. They also showcase novel tools for gaining greater insights into the emotional experience of the participants. These analyses confirm the value, validity, and usability of our annotation framework. The classification results confirmed that ratings patterns were cohesive across the participants. Subsequently, five analyses were undertaken: (i) a System Usability Scale (SUS) questionnaire unveiled the framework's excellent usability (ii) MANOVA analysis of the mean V-A ratings and (iii) trajectory similarity analyses of the annotations confirmed the successful elicitation of emotions (iv) Change point analysis of the annotations, revealed a direct mapping between emotional events and annotations, thereby enabling automatic detection of emotionally salient points in the videos and (v) Support Vector Machines (SVM) were trained on classification of 5 second chunks of annotations as well as their change-points. They were asked to indicate their instantaneous emotional state in a valence-arousal (V-A) space, using a joystick.

To assess the same, 30 subjects each watched 8 emotion-inducing videos.
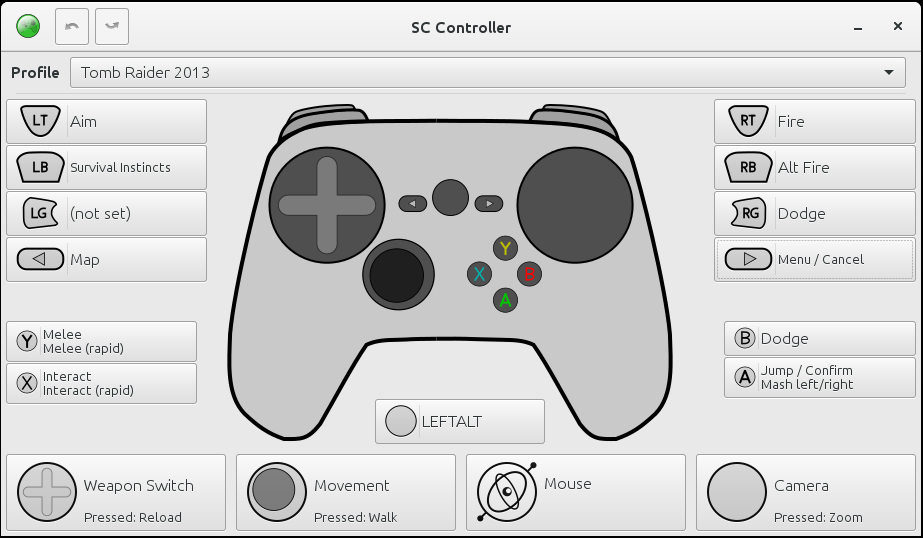
To overcome these limitations, we developed a continuous, real-time, joystick-based emotion annotation framework.
#Sc joystick mapper 2.5 manual#
Emotion labels are usually obtained via either manual annotation, which is tedious and time-consuming, or questionnaires, which neglect the time-varying nature of emotions and depend on human's unreliable introspection.
